Advancing adoptability and sustainability of digital prediction tools for climate-sensitive infectious disease prevention and control
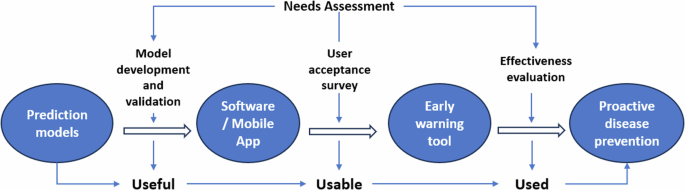
IPCC. Summary for policymakers. In Climate Change 2023: Synthesis Report. Contribution of Working Groups I, II and III to the Sixth Assessment Report of the Intergovernmental Panel on Climate Change (eds Lee, H. & Romero, J) 1–34 (IPCC, Geneva, Switzerland, 2023).
Mordecai, E. A. et al. Thermal biology of mosquito‐borne disease. Ecol. Lett. 22, 1690–1708 (2019).
Google Scholar
Rocklöv, J. & Dubrow, R. Climate change: an enduring challenge for vector-borne disease prevention and control. Nat. Immunol. 21, 479–483 (2020).
Google Scholar
Anas, M. et al. in: Microbiomes and the Global Climate Change (eds Showkat Ahmad Lone & Abdul Malik). 123–144 (Springer, Singapore, 2021).
Alcayna, T. et al. Climate-sensitive disease outbreaks in the aftermath of extreme climatic events: a scoping review. One Earth 5, 336–350 (2022).
Google Scholar
Cascio, W. E. Wildland fire smoke and human health. Sci. Total Environ. 624, 586–595 (2018).
Google Scholar
Reid, C. E. & Maestas, M. M. Wildfire smoke exposure under climate change: impact on respiratory health of affected communities. Curr. Opin. Pulm. Med. 25, 179–187 (2019).
Google Scholar
Mirsaeidi, M. et al. Climate change and respiratory infections. Ann. Am. Thorac. Soc. 13, 1223–1230 (2016).
Google Scholar
Gorris, M. E., Treseder, K. K., Zender, C. S. & Randerson, J. T. Expansion of coccidioidomycosis endemic regions in the United States in response to climate change. GeoHealth 3, 308–327 (2019).
Google Scholar
Head, J. R. et al. Effects of precipitation, heat, and drought on incidence and expansion of coccidioidomycosis in western USA: a longitudinal surveillance study. Lancet Planet. Health 6, e793–e803 (2022).
Google Scholar
He, Y., Liu, W. J., Jia, N., Richardson, S. & Huang, C. Viral respiratory infections in a rapidly changing climate: the need to prepare for the next pandemic. EBioMedicine 93, 104593 (2023).
Google Scholar
Pley, C., Evans, M., Lowe, R., Montgomery, H. & Yacoub, S. Digital and technological innovation in vector-borne disease surveillance to predict, detect, and control climate-driven outbreaks. Lancet Planet. Health 5, e739–e745 (2021).
Google Scholar
Ryan, S. J. et al. The current landscape of software tools for the climate-sensitive infectious disease modelling community. Lancet Planet. Health 7, e527–e536 (2023).
Google Scholar
Neta, G. et al. Advancing climate change health adaptation through implementation science. Lancet Planet. Health 6, e909–e918 (2022).
Google Scholar
Nsubuga, P. et al. Public health surveillance: a tool for targeting and monitoring interventions. In Disease Control Priorities in Developing Countries. 2nd ed. (eds Jamison, D. T. et al.) 997–1015 (Oxford University Press, 2006).
Stewart Ibarra, A. et al. Landscape mapping of software tools for climate-sensitive infectious disease modelling. (Inter-American Institute for Global Change Research: IAI and Wellcome Trust, 2022).
Interaction Design Foundation – IxDF. Useful, Usable, and Used: Why They Matter to Designers. (2020).
Lessler, J., Azman, A. S., Grabowski, M. K., Salje, H. & Rodriguez-Barraquer, I. Trends in the mechanistic and dynamic modeling of infectious diseases. Curr. Epidemiol. Rep. 3, 212–222 (2016).
Google Scholar
Page, M. J. et al. The PRISMA 2020 statement: an updated guideline for reporting systematic reviews. Int. J. Surg. 88, 105906 (2021).
Google Scholar
Lowe, R. et al. Dengue outlook for the World Cup in Brazil: an early warning model framework driven by real-time seasonal climate forecasts. Lancet Infect. Dis. 14, 619–626 (2014).
Google Scholar
Colón-González, F. J. et al. A methodological framework for the evaluation of syndromic surveillance systems: a case study of England. BMC Public Health 18, 1–14 (2018).
Google Scholar
Colón-González, F. J. et al. Probabilistic seasonal dengue forecasting in Vietnam: A modelling study using superensembles. PLoS Med. 18, e1003542 (2021).
Google Scholar
Wikle, C. K. & Zammit-Mangion, A. Statistical deep learning for spatial and spatiotemporal data. Annu. Rev. Stat. Appl. 10, 247–270 (2023).
Google Scholar
Yamana, T. K., Kandula, S. & Shaman, J. Superensemble forecasts of dengue outbreaks. J. R. Soc. Interface 13, 20160410 (2016).
Google Scholar
Johansson, M. A. et al. An open challenge to advance probabilistic forecasting for dengue epidemics. Proc. Natl. Acad. Sci. USA 116, 24268–24274 (2019).
Google Scholar
Hyndman, R. J. & Athanasopoulos, G. Forecasting: Principles and Practice. 3rd edition. (OTexts, 2018).
Bürkner, P.-C., Gabry, J. & Vehtari, A. Approximate leave-future-out cross-validation for Bayesian time series models. J. Stat. Comput. Simul. 90, 2499–2523 (2020).
Google Scholar
Funk, S. et al. Assessing the performance of real-time epidemic forecasts: A case study of Ebola in the Western Area region of Sierra Leone, 2014-15. PLoS Comput. Biol. 15, e1006785 (2019).
Google Scholar
Brier, G. W. Verification of forecasts expressed in terms of probability. Mon. Weather Rev. 78, 1–3 (1950).
Google Scholar
Bradshaw, J. in Problems and Progress in Medical Care: Essays on Current Research, 7th series (ed Gordon McLachlan). 71–82 (Oxford, Oxford University Press, 1972).
Holden, R. J. & Karsh, B.-T. The technology acceptance model: its past and its future in health care. J. Biomed. Inform. 43, 159–172 (2010).
Google Scholar
Davis, F. D., Bagozzi, R. P. & Warshaw, P. R. User acceptance of computer technology: A comparison of two theoretical models. Manag. Sci. 35, 982–1003 (1989).
Google Scholar
Venkatesh, V. & Davis, F. D. A theoretical extension of the technology acceptance model: Four longitudinal field studies. Manag. Sci. 46, 186–204 (2000).
Google Scholar
Tamilmani, K., Rana, N. P., Wamba, S. F. & Dwivedi, R. The extended Unified Theory of Acceptance and Use of Technology (UTAUT2): A systematic literature review and theory evaluation. Int. J. Inf. Manage. 57, 102269 (2021).
Google Scholar
Royse, D., Staton-Tindall, M., Badger, K. & Webster, J. M. Needs Assessment. (Oxford: Oxford University Press, 2009).
Bradshaw, J. The conceptualization and measurement of need: a social policy perspective. In Researching the People’s Health (eds Popay, J & Williams, G) 45–57 (Routledge, London, 1994).
Hayes, R. J. & Moulton, L. H. Cluster Randomised Trials. (Boca Raton, Florida, Chapman and Hall/CRC, 2017).
Hayes, R., Alexander, N. D., Bennett, S. & Cousens, S. Design and analysis issues in cluster-randomized trials of interventions against infectious diseases. Stat. Methods Med. Res. 9, 95–116 (2000).
Google Scholar
Andersson, N. et al. Evidence based community mobilization for dengue prevention in Nicaragua and Mexico (Camino Verde, the Green Way): cluster randomized controlled trial. BMJ 351, h3267 (2015).
Google Scholar
Dabira, E. D. et al. Mass drug administration of ivermectin and dihydroartemisinin–piperaquine against malaria in settings with high coverage of standard control interventions: a cluster-randomised controlled trial in The Gambia. Lancet Infect. Dis. 22, 519–528 (2022).
Google Scholar
Cha, S. et al. Effect of a community-led total sanitation intervention on the incidence and prevalence of diarrhea in children in rural Ethiopia: a cluster-randomized controlled trial. Am. J. Trop. Med. Hyg. 105, 532 (2021).
Google Scholar
Hurley, J. C. How the cluster-randomized trial “works. Clin. Infect. Dis. 70, 341–346 (2020).
Google Scholar
Sanders, G. D. et al. Recommendations for conduct, methodological practices, and reporting of cost-effectiveness analyses: second panel on cost-effectiveness in health and medicine. JAMA 316, 1093–1103 (2016).
Google Scholar
Cavany, S. et al. Does ignoring transmission dynamics lead to underestimation of the impact of interventions against mosquito-borne disease? BMJ Glob. Health 8, e012169 (2023).
Google Scholar
Eliopoulos, G. M. et al. The use and interpretation of quasi-experimental studies in infectious diseases. Clin. Infect. Dis. 38, 1586–1591 (2004).
Google Scholar
Brazil Ministério da Saúde. Atualização de Casos de Arboviroses. (2024).
Alemayehu, C., Mitchell, G. & Nikles, J. Barriers for conducting clinical trials in developing countries- a systematic review. Int. J. Equity Health 17, 37 (2018).
Google Scholar
Kuhn, K. Campbell-Lindrum, D., Haines, A. & Cox, J. Using Climate to Predict Infectious Disease Epidemics. (Geneva: World Health Organization, 2005).
link