The future of digital health with federated learning

ML and especially DL is becoming the de facto knowledge discovery approach in many industries, but successfully implementing data-driven applications requires large and diverse data sets. However, medical data sets are difficult to obtain (subsection “The reliance on data”). FL addresses this issue by enabling collaborative learning without centralising data (subsection “The promise of federated efforts”) and has already found its way to digital health applications (subsection “Current FL efforts for digital health”). This new learning paradigm requires consideration from, but also offers benefits to, various healthcare stakeholders (section “Impact on stakeholders”).
The reliance on data
Data-driven approaches rely on data that truly represent the underlying data distribution of the problem. While this is a well-known requirement, state-of-the-art algorithms are usually evaluated on carefully curated data sets, often originating from only a few sources. This can introduce biases where demographics (e.g., gender, age) or technical imbalances (e.g., acquisition protocol, equipment manufacturer) skew predictions and adversely affect the accuracy for certain groups or sites. However, to capture subtle relationships between disease patterns, socio-economic and genetic factors, as well as complex and rare cases, it is crucial to expose a model to diverse cases.
The need for large databases for AI training has spawned many initiatives seeking to pool data from multiple institutions. This data is often amassed into so-called Data Lakes. These have been built with the aim of leveraging either the commercial value of data, e.g., IBM’s Merge Healthcare acquisition21, or as a resource for economic growth and scientific progress, e.g., NHS Scotland’s National Safe Haven22, French Health Data Hub23, and Health Data Research UK24.
Substantial, albeit smaller, initiatives include the Human Connectome25, the UK Biobank26, the Cancer Imaging Archive (TCIA)27, NIH CXR828, NIH DeepLesion29, the Cancer Genome Atlas (TCGA)30, the Alzheimer’s Disease Neuroimaging Initiative (ADNI)31, as well as medical grand challenges32 such as the CAMELYON challenge33, the International multimodal Brain Tumor Segmentation (BraTS) challenge34,35,36 or the Medical Segmentation Decathlon37. Public medical data is usually task- or disease-specific and often released with varying degrees of license restrictions, sometimes limiting its exploitation.
Centralising or releasing data, however, poses not only regulatory, ethical and legal challenges, related to privacy and data protection, but also technical ones. Anonymising, controlling access and safely transferring healthcare data is a non-trivial, and sometimes impossible task. Anonymised data from the electronic health record can appear innocuous and GDPR/PHI compliant, but just a few data elements may allow for patient reidentification7. The same applies to genomic data and medical images making them as unique as a fingerprint38. Therefore, unless the anonymisation process destroys the fidelity of the data, likely rendering it useless, patient reidentification or information leakage cannot be ruled out. Gated access for approved users is often proposed as a putative solution to this issue. However, besides limiting data availability, this is only practical for cases in which the consent granted by the data owners is unconditional, since recalling data from those who may have had access to the data is practically unenforceable.
The promise of federated efforts
The promise of FL is simple—to address privacy and data governance challenges by enabling ML from non-co-located data. In a FL setting, each data controller not only defines its own governance processes and associated privacy policies, but also controls data access and has the ability to revoke it. This includes both the training, as well as the validation phase. In this way, FL could create new opportunities, e.g., by allowing large-scale, in-institutional validation, or by enabling novel research on rare diseases, where the incident rates are low and data sets at each single institution are too small. Moving the model to the data and not vice versa has another major advantage: high-dimensional, storage-intense medical data does not have to be duplicated from local institutions in a centralised pool and duplicated again by every user that uses this data for local model training. As the model is transferred to the local institutions, it can scale naturally with a potentially growing global data set without disproportionately increasing data storage requirements.
As depicted in Fig. 2, a FL workflow can be realised with different topologies and compute plans. The two most common ones for healthcare applications are via an aggregation server16,17,18 and peer to peer approaches15,39. In all cases, FL implicitly offers a certain degree of privacy, as FL participants never directly access data from other institutions and only receive model parameters that are aggregated over several participants. In a FL workflow with aggregation server, the participating institutions can even remain unknown to each other. However, it has been shown that the models themselves can, under certain conditions, memorise information40,41,42,43. Therefore, mechanisms such as differential privacy44,45 or learning from encrypted data have been proposed to further enhance privacy in a FL setting (c.f. section “Technical considerations”). Overall, the potential of FL for healthcare applications has sparked interest in the community46 and FL techniques are a growing area of research12,20.
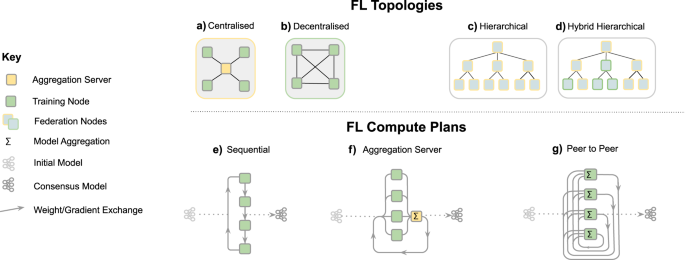
FL topologies—communication architecture of a federation. a Centralised: the aggregation server coordinates the training iterations and collects, aggregates and distributes the models to and from the Training Nodes (Hub & Spoke). b Decentralised: each training node is connected to one or more peers and aggregation occurs on each node in parallel. c Hierarchical: federated networks can be composed from several sub-federations, which can be built from a mix of Peer to Peer and Aggregation Server federations (d)). FL compute plans—trajectory of a model across several partners. e Sequential training/cyclic transfer learning. f Aggregation server, g Peer to Peer.
Current FL efforts for digital health
Since FL is a general learning paradigm that removes the data pooling requirement for AI model development, the application range of FL spans the whole of AI for healthcare. By providing an opportunity to capture larger data variability and to analyse patients across different demographics, FL may enable disruptive innovations for the future but is also being employed right now.
In the context of electronic health records (EHR), for example, FL helps to represent and to find clinically similar patients13,47, as well as predicting hospitalisations due to cardiac events14, mortality and ICU stay time19. The applicability and advantages of FL have also been demonstrated in the field of medical imaging, for whole-brain segmentation in MRI15, as well as brain tumour segmentation16,17. Recently, the technique has been employed for fMRI classification to find reliable disease-related biomarkers18 and suggested as a promising approach in the context of COVID-1948.
It is worth noting that FL efforts require agreements to define the scope, aim and technologies used which, since it is still novel, can be difficult to pin down. In this context, today’s large-scale initiatives really are the pioneers of tomorrow’s standards for safe, fair and innovative collaboration in healthcare applications.
These include consortia that aim to advance academic research, such as the Trustworthy Federated Data Analytics (TFDA) project49 and the German Cancer Consortium’s Joint Imaging Platform50, which enable decentralised research across German medical imaging research institutions. Another example is an international research collaboration that uses FL for the development of AI models for the assessment of mammograms51. The study showed that the FL-generated models outperformed those trained on a single institute’s data and were more generalisable, so that they still performed well on other institutes’ data. However, FL is not limited just to academic environments.
By linking healthcare institutions, not restricted to research centres, FL can have direct clinical impact. The on-going HealthChain project52, for example, aims to develop and deploy a FL framework across four hospitals in France. This solution generates common models that can predict treatment response for breast cancer and melanoma patients. It helps oncologists to determine the most effective treatment for each patient from their histology slides or dermoscopy images. Another large-scale effort is the Federated Tumour Segmentation (FeTS) initiative53, which is an international federation of 30 committed healthcare institutions using an open-source FL framework with a graphical user interface. The aim is to improve tumour boundary detection, including brain glioma, breast tumours, liver tumours and bone lesions from multiple myeloma patients.
Another area of impact is within industrial research and translation. FL enables collaborative research for, even competing, companies. In this context, one of the largest initiatives is the Melloddy project54. It is a project aiming to deploy multi-task FL across the data sets of 10 pharmaceutical companies. By training a common predictive model, which infers how chemical compounds bind to proteins, partners intend to optimise the drug discovery process without revealing their highly valuable in-house data.
Impact on stakeholders
FL comprises a paradigm shift from centralised data lakes and it is important to understand its impact on the various stakeholders in a FL ecosystem.
Clinicians
Clinicians are usually exposed to a sub-group of the population based on their location and demographic environment, which may cause biased assumptions about the probability of certain diseases or their interconnection. By using ML-based systems, e.g., as a second reader, they can augment their own expertise with expert knowledge from other institutions, ensuring a consistency of diagnosis not attainable today. While this applies to ML-based system in general, systems trained in a federated fashion are potentially able to yield even less biased decisions and higher sensitivity to rare cases as they were likely exposed to a more complete data distribution. However, this demands some up-front effort such as compliance with agreements, e.g., regarding the data structure, annotation and report protocol, which is necessary to ensure that the information is presented to collaborators in a commonly understood format.
Patients
Patients are usually treated locally. Establishing FL on a global scale could ensure high quality of clinical decisions regardless of the treatment location. In particular, patients requiring medical attention in remote areas could benefit from the same high-quality ML-aided diagnoses that are available in hospitals with a large number of cases. The same holds true for rare, or geographically uncommon, diseases, that are likely to have milder consequences if faster and more accurate diagnoses can be made. FL may also lower the hurdle for becoming a data donor, since patients can be reassured that the data remains with their own institution and data access can be revoked.
Hospitals and practices
Hospitals and practices can remain in full control and possession of their patient data with complete traceability of data access, limiting the risk of misuse by third parties. However, this will require investment in on-premise computing infrastructure or private-cloud service provision and adherence to standardised and synoptic data formats so that ML models can be trained and evaluated seamlessly. The amount of necessary compute capability depends of course on whether a site is only participating in evaluation and testing efforts or also in training efforts. Even relatively small institutions can participate and they will still benefit from collective models generated.
Researchers and AI developers
Researchers and AI developers stand to benefit from access to a potentially vast collection of real-world data, which will particularly impact smaller research labs and start-ups. Thus, resources can be directed towards solving clinical needs and associated technical problems rather than relying on the limited supply of open data sets. At the same time, it will be necessary to conduct research on algorithmic strategies for federated training, e.g., how to combine models or updates efficiently, how to be robust to distribution shifts11,12,20. FL-based development implies also that the researcher or AI developer cannot investigate or visualise all of the data on which the model is trained, e.g., it is not possible to look at an individual failure case to understand why the current model performs poorly on it.
Healthcare providers
Healthcare providers in many countries are affected by the on-going paradigm shift from volume-based, i.e., fee-for-service-based, to value-based healthcare, which is in turn strongly connected to the successful establishment of precision medicine. This is not about promoting more expensive individualised therapies but instead about achieving better outcomes sooner through more focused treatment, thereby reducing the cost. FL has the potential to increase the accuracy and robustness of healthcare AI, while reducing costs and improving patient outcomes, and may therefore be vital to precision medicine.
Manufacturers
Manufacturers of healthcare software and hardware could benefit from FL as well, since combining the learning from many devices and applications, without revealing patient-specific information, can facilitate the continuous validation or improvement of their ML-based systems. However, realising such a capability may require significant upgrades to local compute, data storage, networking capabilities and associated software.
link